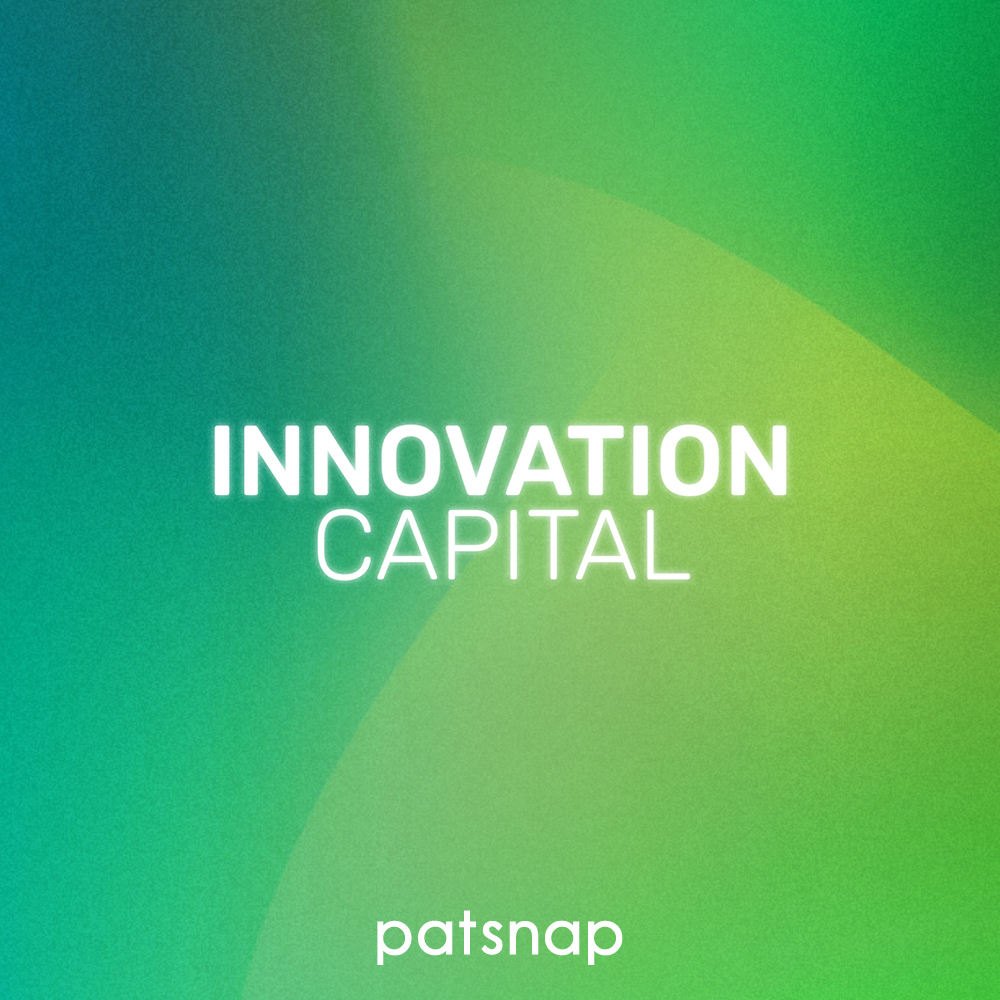
Episode 3 of PatSnap's Innovation Capital podcast
Digital R&D – Innovation Process with AI, ft. Kevin See
About Innovation Capital
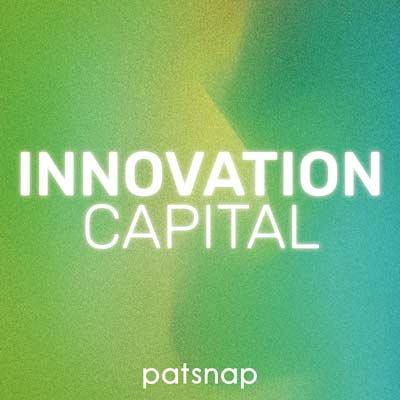
Inspired by the words of U.S. inventor Charles Kettering, “if you have always done it that way, it’s probably wrong,” Innovation Capital, presented by PatSnap, was born out of a desire to go where no other innovation podcast has gone. Just as the world’s top innovators have pushed the boundaries of what’s familiar and accepted, host Ray Chohan takes a completely fresh and unfiltered look at some of the biggest topics shaping innovation today. From the key drivers of innovation, to its role in the economic value chain and groundbreaking outputs, Innovation Capital leaves no question unanswered. When it comes to innovation, we are your capital; your mecca for daring discussion and the fuel for your growth and scalability. Welcome to Innovation Capital.
Subscribe to Innovation Capital:
In this episode of Innovation Capital
We focus on the impact of AI as a method of invention that can reshape the nature of the innovation process and the organization of R&D. Through an exploration of how AI automates innovation and discovery, we will uncover its game-changing benefits, including the decreased cost of doing R&D in many fields, an increase in research productivity, and the creation of more value for consumers and shareholders.
Listen now
Episode highlights
- The impact of AI is being felt in the later stages of the innovation funnel.
- Some large corporations are experimenting with topic modeling and NPL in the front end of the lifecycle, primarily for drug discovery purposes.
- Data science is finding a foothold in the manufacturing sector, supply chain and CPG world where AI is being used to better understand customers and integrate those insights into their innovation flow.
- The vast majority of companies are not well positioned to leverage the amount of data they have.
- The smartest approach is to pinpoint the problem you want AI to solve, look for small wins first, and “nail it before you scale it.”
- Get our #1 Amazon bestselling eBook, The Definitive Guide to Connected Innovation Intelligence (CII). In this white paper, we explore what CII is, who it’s for, and how the world’s disruptors are using it to win in hyper-competitive markets. Download your FREE copy.
The experts
-
Episode Guest:
Kevin See
Vice President of Research, Lux Research
As VP of Research at Lux Research, Kevin is responsible for the development and deployment of technologies to support research products within Lux, and for providing solutions externally to clients in the digital space. Prior to joining Lux, Kevin was a joint postdoctoral researcher at The Molecular Foundry at Lawrence Berkeley National Laboratory and The University of California, Berkeley. Kevin obtained his PhD. in Materials Science and Engineering from Johns Hopkins University and has authored articles in leading journals on subjects including nanocomposites, organic electronics, sensors, and thermoelectrics.
-
Host:
Ray Chohan
Founder West & VP New Ventures, PatSnap
Ray is Founder West & VP New Ventures and the founding member of PatSnap in Europe. He started the London operation from his living room in 2012, growing the team to 70+ by 2015. Prior to PatSnap, Ray was BD Director at Datamonitor where he was an award-winning revenue generator across various verticals and product lines over an 8-year period. This journey gave Ray the unique insight and inspiration to start the PatSnap ‘go to market’ in London. Ray now leads corporate development where he focuses his time on creating new partnerships and go-to-market strategies.
Episode transcript
Ray Chohan: So Kevin, welcome to Innovation Capital. It’s great to have you here today. I would love to kick off with a little bit of your story and how you ended up at Lux Research, and became one of the preeminent thought leaders within AI and R&D?
Kevin See: Yeah, thanks, Ray. Appreciate the invitation and happy to be here. My background is, I’m trained as a scientist. I did a PhD and a postdoc largely focused on developing material devices for a variety of applications, from sensors to energy harvesting. So, I was deep in the lab, designing, building and testing. At that point, after I wrapped up that academic portion, I was really interested in the commercialization pathway for emerging technologies. Some of the things that I saw my peers working on in the lab, but I also had the sneaking suspicion that a lot of these projects might not have a lot of promise to them. So, I became very interested in what does it take for technology commercialization to be successful, and ultimately create some value for somebody. So, that interest led me to Lux, which is really an ideal place to foster some of those interests. We really value the technology strength, but also marry that with some business understanding of what it takes to actually commercialize things. So, from there, I’ve been part of just scaling our business model to look at everything from energy technologies, renewables, expanded us into things like digital and AI, which we’re going to talk about today. I had a stint helping lead our products, which was really developing and internalizing AI capabilities ourselves. So, just a variety of roles that were a lot of fun.
Ray: Looking at 2020 and beyond, we have a sentiment that we’re entering, hopefully, a glorious era of how machine learning in particular will really impact the innovation process. But, before we look at more of a forward-looking outlook, in the next five, six years, I would love to get your professional insight on the last decade, and how we’ve led up to the stage where we’re going to enhance the digital journey within R&D and innovation. Do you have some background context, some kind of historical tailwinds, which led us to where we are today, Kevin?
Kevin: Yeah, I think that’s a good question, Ray. I think that largely depends on how you define digital. Digital can mean a lot of things. It could mean AI for discovery, which is maybe the sexy version of it. But it could also be more operational things like just tools to manage your innovation pipeline and things like that. So, I’d say across the board, we’re still in the pretty early stages. There’s definitely been advances, there’s definitely been pilots. They try new things but these grand ambitions of automating inside the R&D process, largely, no one’s really there. You see people trying isolated pilots, and different things like that. You do see other digital tools, which is a little bit more advanced, which is just really managing knowledge or managing pipeline of ideas. So again, depending on how you really define digital, you know, is it this aggressive, world-changing approach? Or is it sometimes mundane? Managing a process? It really depends on how you look at it. But, I really think we are only now kind of entering into that phase where we’ll start to see some of these more disruptive applications of digital.
Ray: Is it fair to say we are in the first innings? And if we are not, where are we on that journey in terms of getting there?
Kevin: I’d say on that particular aspect, we’re really early days. We see people again, trying pilots, trying machine learning, you know; How do I find interesting papers that are associated with things that I’m interested in? You see things like that starting to occur, but in terms of really scaling that like signal detection and idea generation, in an automated means or informed data or analytics, I’d say we’re really quite in the early stages there. And we haven’t seen anyone really push that, that fuzzy front end, forward really aggressively just yet.
Ray: So, if you were to use more of a, say, the start of the internet analogy in the 90s, what year are we in when it comes to really machine learning? And really revolutionizing that that fuzzy front end, Kevin?
Kevin: Yeah, in terms of that front end, I’d say, I mean, this is a little bit of a tell on how old I am. But basically, I remember getting to University and, you know, it’s really the onset of the Ethernet connections and the T1 just opening up our eyes to the power of the internet. And it was really uncharted territory. I think that’s probably a good analog for where we are now in terms of really taking a pretty established process in terms of ideation on that fuzzy front end, just exploring the possibilities, really knowing that the tools are there, but not quite really understanding how to use them or what they can do. So, I’d say it’s definitely early days there.
Ray: Oh, okay. Kevin, you’re bringing back some memories, my friend with T1 connection. So, that’s some good context for some people who maybe are older listeners. So really, we’re at like, maybe 96′ 97′ in terms of maturity. Is that fair for us to say?
Kevin: Yeah. I would say that that’s a fair analogue in terms of timeline.
Ray: Brilliant. And in terms of some of the trailblazers, Kevin, because I’ve seen some of your work, highlighting some interesting case studies where there’s a few organizations really moving the needle on how AI, machine learning, deep neural nets, are really supporting that innovation process, be it the fuzzy front end, or later in the cycle. Have you got some favourite case studies, which you think have really sent a ripple in the market?
Kevin: Yeah, I think not so much, there’s a plethora of players on that ideation and front-end part of the funnel that we’re discussing. There’s definitely players that are emerging. They’re doing interesting work. I think both of our firms are doing interesting work there. But, I think where you see more maturity is further down that funnel, which is more into something like materials informatics, where using a ton of data at your disposal to try and figure out, What do I make next that will have the properties that I want? So, we’ve seen in our research and the work we do a lot of interesting companies. The one that pops out is someone like Citrine Informatics, doing some interesting work there. But in terms of that ideation front end part, I’d say it’s still a bit of an open playing field, really trying out how to develop the right tools that are useful for the scientists on the ground. I think there’s a ton of interesting work there. But I wouldn’t say that, you know, the singular leader has emerged yet.
Ray: It’s fascinating. You mentioned the materials informatics space, it’s an area that we’re deeply passionate about. And yeah, we love what Citrine and a couple of other folks in the Boston area are doing in that space. So, on a broader sense, is it fair to say you’re seeing more progression farther up in the funnel? So when we get into maybe the project lifecycle management space, where it’s going more deeper into the workflow, where you’re seeing the impact of AI, more than at the development and kind of launch phase?
Kevin: Yeah, again, it depends on how you think about it. I would say that you’re right, if you put materials informatics, depending on how you define, you know, the stage of the funnel, it is a little bit further, it’s still early stage, but you’re moving past that ideation phase into actual discovery, you know, what is the thing I’m going to work on, and pushing that forward more aggressively. So, I think there’s definitely more happening there. I think if you go back to things like project management and lifecycle, you know those things don’t really require AI necessarily. So, I think it’s a case where certainly it’s digital but, you know, to manage a pipeline of companies or manage a pipeline of projects more efficiently, you don’t really need AI necessarily. So, it’s just you know, what aspect of the funnel you’re at, which tools are you most interested in? And I guess we’ll probably come back to this but I think something that’s important is that AI is not always the right tool. You don’t always need AI to solve all your problems. I think that’s something to keep in mind that there’s low hanging fruit as well.
Ray: It’s interesting, and you publish some fascinating research talking about topic modeling, NLP and how it has the potential to really move the needle on that fuzzy front end. But in reality, when you look in market, it’s pretty much under utilized at the moment. Do you think there’s been an accelerant this year, especially with COVID, and R&D being done remote more than ever. Are there any events that have occurred in 2020 where you see an accelerant regarding topic modeling and an overlap on content?
Kevin: Yeah, I haven’t really seen COVID necessarily as an enabler or an accelerant. At that very, you know, we call weak signal detection or early detection of ideas. You have seen it, obviously, in some of them, the big obvious things like vaccines, or drug discovery, which again, is a little bit further down. It’s not in the wide open playing field that signal detection is, where you’re just looking for, What can I possibly work on? And again, some of these pockets of pilots, with something like NLP, or a topic model or a classifier is, you know, a research group or a company finds a paper of interest, they can actually use some of these AI tools to say; What are other papers like this, that are worth me looking into, that should be relevant based on my interest in this one topic? So, that’s where you can do some recognition of what’s interesting to you. We have heard of some larger corporate players and others, starting to play around with those tools a little more, I wouldn’t say it’s been built into the larger infrastructure just yet but you do see people exploring some of those tools. I don’t think the events of 2020 have necessarily accelerated that particular use, I think it’s just this evolution where particularly corporations are becoming more sophisticated about AI and they’re starting to poke around to try and understand how they can use it.
Ray: And from the customer end, what are you seeing what are you seeing in terms of philosophy? Is it build, home grow? Or buy or partner? What are you seeing certain sectors go down? Because we see a mixture, but we’d love to get your lens.
Kevin: Ya, we see a mix as well. I think this is where it can be a pretty sticky situation for a lot of corporations. Because their strength isn’t necessarily in building. It’s really almost building enterprise software for scientists and you know that well, right. But it’s challenging. It’s a very demanding use case and audience. And so what you see is corporation sometimes have their IT department brought in to develop software, tools, and then sometimes the user interface or other things are just not very good. And it’s hard to get traction for them internally. So, we believe that there’s value in probably bringing internal expertise to bear. You know, your customers essentially, in that case, they know that very well. But they’re not always the best equipped to deliver those solutions. So, we’ve seen some missteps, where you build stuff that people don’t use, largely because of that. It does makes sense to buy in this case, or partner. And it can be a variety of different kinds of partners. There’s huge enterprises that could help you build it, there’s emerging players but the key part is that you’re looking at a mixture of AI and data science data science capabilities, that firstly, not every company has; in fact, that skill set is in huge demand. And it’s hard to find strong people there. But you’re also building software in many cases. So, it’s not just doing data science in the back, crunching data and just spitting out results. It’s actually building interfaces and things that people have to use. And, again, these aren’t necessarily things corporations in various industries are good at. So, to that extent we’ve tried to push people towards our clients who asked about it. To me personally, I think it does make sense to get that expertise from partners and make sure you integrate it with the knowledge that exists internally.
It’s fascinating. You mentioned that momentum around how enterprises, large funds, are trying to skate out their data science effort and scale that headcount?
Ray: Where are you seeing some of the hyper growth in terms of industries or sub sectors where they’re really pushing the agenda on data science, decision science, and trying to really ramp up that internal capability of it?
Kevin: Yeah, it’s a huge, huge effort. It is really around industry for manufacturing. Just to some extent, it’s a well-known optimization problem. So, throwing data science at things like predictive maintenance, or quality or other things just makes sense. It’s something they understand in terms of a need or a use case. They can bring data science, to innovate within that specific set of use cases whether it’s supply chain manufacturing. You can think about it also as an innovation funnel, not necessarily as idea turning into product. But in an instance, like that, it’s the idea of turning into deployment, or operational use internally. But you do see data scientists in demand for that application, because it’s a well known problem, it’s well understood, and there can be an immediate benefit to the organization that they can measure in terms of dollars. So that’s definitely a place where we see a kind of a clamoring to bring on that data science talent.
Ray: And looking forward, where do you see some of the blue oceans where data science is going to make a meaningful impact in that Stage-Gate process, or generally that the overall R&D and innovation process?
Kevin: Yeah, I think that materials discovery, for example, you know, essentially taking the learnings from the pharma industry, which has been a bit more advanced in terms of AI for things like drug discovery importing that into other analogous type of industries; like developing a polymer with a certain set of properties. I think that’s also an area that’s near and dear to my heart from a background perspective. But, I think that it’s tremendously impactful to leverage all the articles, all the papers, all the results from experiments. If you can structure and process that data, you can turn that into really accelerating the discovery and design and production of things with the properties that you want. So, that’s something that I think is a particularly interesting application of AI.
On a totally different front. I think there’s a lot of interest in the CPG world, or the consumer goods world, where you can actually use AI, to better understand your end user (in this case, the customer) and trickle that back into your, your innovation funnel. So, what are the trends I’m seeing in my customer base? What are the different data sets I can pull together? What are the patterns that I can pull out? How can that better inform the products that I design next? So, you see kind of a virtuous circle there, where you design products extensively with some measure of AI and IoT embedded that gathers more data about the customer, you feed that back in. So, you see this capability of really understanding your end user and customer better. I think that can only make that whole product development cycle more efficient and more effective.
Ray: And it’s funny, you mentioned the materials informatics space a couple of times, and that’s an area which is very much close to our heart as well. Is that a compelling area in terms of ML really moving the needle for material science teams across different industries? Are you getting good sentiment and feedback, where the buyers or that type of capability, kind of really get it on our board?
Kevin: Yeah, I’d say if you’re talking to the right person at the right company. So obviously, in this case, we’re talking about the chemicals and materials sector. They know how hard it is to design the next product, whether it’s a small molecule or whether it’s a composite or a polymer. And I’ve been in the lab trying to design these things myself and it’s hard and there is trial and error and inference. And so there’s definitely an understanding that if it works, which is a big if, the ability to sift through all the existing data to better inform where I should put my resources is a huge value add to a corporation. It can speed up time to delivering a product, cut down on R&D costs, lots of benefits there. So, I do think it’s a tough customer base. They’ve done things one way for a long time. But we do see increasing appetite to use digital tools to try and facilitate, accelerate that particular use case. Because if you get it right, it’s great, but it’s probably not quite the same scale as drug discovery. It is a pretty huge value add if you can get to profitable products faster. So that’s the thing that we see people being really attracted to.
Ray: Yeah, it’s interesting, you segued into the drug discovery space. Again, we’re seeing some brilliant developments in that area for the last 24 months in particular, from the market, really seeing that philosophical buying and that eagerness to deploy ML at the bench level, at the discovery stage, and further up the stage in the drug development cycle. What are your thoughts on some of the low hanging fruit in that area in the next two or three years? Are there some great examples which really catch your eye? And where do you see some of the compelling growth opportunities when you look at AI-driven intelligent drug discovery?
Kevin: Yeah, I’d say, this is not the space I spend most of my time looking at, but in terms of observing the market and seeing the things like Moderna, and how much they really emphasize digital and AI as part of their overall corporate strategy, in building the company. And that’s obviously seen fruits in the vaccine development and other things. So, that’s an acute example of where COVID showed real value for digitalizing that discovery process. So, there’s some, you know, acute, obvious ones like that. Overall, I think there’s just such a vast set of data that they’re sitting on as an industry or as a sector. So, genetic data, which is just only becoming easier to acquire, easier to test for and synthesize. Those types of datasets plus clinical outcomes, this idea of where you can really correlate things far upstream with outcomes in the patient. There’s just a tremendous amount of opportunity and leveraging that going forward. I would be hard pressed to pick one particular application for it, because I think it’s so widespread, but I really do think I’m attracted to that particular problem just because of how vast that data is. And we’ll probably get into this, but really, the quality of the insights you get out really depend on the data you put in. And I think there’s just so much of it that drug discovery is pretty ripe for using AI.
Ray: It’s fascinating, you mentioned, that data quality piece, and that whole rigor around normalization. We hear that sentiment a lot. I mean, building an ML model is one thing, but getting your house in order and actually having the boring stuff, your data operations set up in a best-in-class fashion. Where do you see organizations on that front? Do you think the enterprise is actually truly ready to really optimize the value from machine learning and subsectors of ML, like NLP and topic modeling? Or is it still a journey?
Kevin: It’s definitely still a journey. And I would say the vast majority of companies are not well positioned to leverage the data they have. That’s why they run into problems bringing in a partner or vendor to help them plug data into my black box and turn out valuable insights. They realize very quickly, that if the quality of that data going in is bad, or poorly structured, poorly labeled, and so on, so are the results. So, dirty data equals dirty results. And I think that’s where the frustration comes in where everybody wants to use AI, but doesn’t necessarily recognize the amount of work it takes to prepare yourself from a data perspective to get good results back out. And I would in my experience, talking with corporations, or others who are interested in using some of these tools, like NLP, is just generally running into a bottleneck with the data inputs to do it well. So, a lot of cost has to be assigned, and prepared for, into just cleaning up the data sets you have, whether it’s lab notebooks, or whether it’s external data, like papers, you know, labeling it and structuring it as best you can. Huge issues. And I do think it’s a major bottleneck.
Ray: Interesting, you mentioned that. So, there’s a group out in the Bay Area, called scale.ai. And they’re doing some really fascinating work with all the big auto manufacturers on getting their house in order on enabling the journey to autonomous vehicles, especially some of the traditional players outside of Tesla. So, we are seeing a few companies really being a great partner on that front. But looking at other industries, you’re looking at the chemical space or even pharma or FMCG, or, or the IT space. What is the actual problem on the data side? Is it exec buy-in? Is it just legacy infrastructure and plumbing? What are things that needs to be done, do you think, in the next couple of years to have those organizations optimized on a data cleanliness front to really optimize the value from ML?
Kevin: So that almost speaks to some of the dysfunctions you can see in large organizations in terms of truly understanding the problem to be solved, and what it takes to solve it. And that’s not endemic just to large corporations. That’s a problem, I think, for lots of organizations. But I do think there’s not always transparency, when these initiatives are messaged or launched, really just how hard they’re going to be, and just what level of results you can expect out of them. So, I believe and have seen a lot of initiatives launched, without really a lot of due diligence, necessarily about how hard it’s going to be or what outcomes that are unexpected. You know, in the most extreme case, it’s ‘let’s use AI, because it’s AI, and see what happens.’ And, you know, I see, increasingly people are becoming more sophisticated than that. But certainly in the early “quote, unquote,” digital transformation days, you did not see a lot of rigor, go into choosing the problems and choosing the right tools. So, when you bring up the data problem, you’re basically seeing organizations not really unified and understanding what it’s going to take to get those good results back out, like I talked about before. I think that requires really understanding the true cost. What’s the cost? And really the cost benefit? So, what is the work I’m going to have to put in, I can bring in sexy vendor x, but how much work? And how much are they going to charge me just to clean up my own data, before I can even start to put it into the black box and start to get results out? So, I think there’s just often a lack of understanding exactly how challenging that’s going to be. You pointed out, it could be purely where that data resides, it could be unifying data sets from different parts of a system or different parts of an organization, all kinds of infrastructure issues. So, that data management part is more challenging than people often understand.
Ray: Is there any companies or industries you think are really moving the needle on that front? Who are improving that readiness where they can really truly ingest an AI black box and really optimize the value. Are their sectors that catch your eye who are potential trailblazers on that front?
Kevin: Yeah, it’s gonna sound repetitive, but I do think manufacturing is a place where you start to see people focused on pulling data out of different systems and unifying it. So, I won’t go into all the players necessarily here but I think there is an understanding of my infrastructure has equipment from all these different vendors and just having a way to plug in to All those different systems and put all that data in one place, can let me do a lot more interesting things with it, ultimately, whether it’s AI or other analytics approaches. So, again, that’s one particular place where I see people understanding the challenge of the problem, but also starting to move towards solving it by having the right connectors to pull out the right data. But again, that’s pretty far down the application funnel, compared to looking at journal articles or something for R&D. Right, those are pretty different use cases.
Ray: When you do have the data operations in order and you see an enterprise who do have quality rigor around that, are you seeing true value being realized? Because we see some interesting moments where some subsectors really realize the value, and then some have a lot of hype, but don’t really realize meaningful value for AI being deployed in R&D? What are some of the results you’re seeing in the market in terms of true impact?
Kevin: Yeah, besides the case studies, you know, these big clear values like vaccine discovery or something like we talked about before, in most traditional physical industries, it’s non-pharma based. I haven’t seen this being a huge win from AI yet. I think that’s not because it can’t, but I do think there is just these organizational barriers. Again, it comes down to are you picking the right problem? Are you picking the right tool? Are you getting small wins before you get your big wins? I think there’s a way to build up to some of these more enterprise-wide solutions that involve winning at a small scale. And I don’t know how systematic people have been about identifying those small wins first, and really optimizing or increasing their chance of success. Because you got to win small before you win big, particularly in this case, I think. So, I do think there’s definite room to really think about the problems you’re trying to solve. You know that that example I gave where I want to find papers like this one, you know, not huge in terms of executing, but maybe pretty decent return on investment, if you actually do it in a way that scientists can focus on the top 10 papers. So, I think there’s a way to be more thoughtful about the scale of the problem, you choose what you’re trying to solve, and really being thoughtful about that.
Ray: We see that as well, Kevin. There’s a lot of hype, where people try boiling the ocean. And there isn’t much deep first-principles thinking around, let’s look at a pointed problem and let’s do that well, and kind of land and expand from there. So, it’s fascinating, you mentioned that, because we also hear that sentiment in the market, where folks are really enthusiastic, they get it, they do understand the potential fundamental value, but there is misalignment around taking things in baby steps. So, that there’s confusion around, kind of, nail it before you scale it, around AI being deployed in the innovation process. What do you think it comes down to really, if you really look at it in its first principles, is it the people? Is it the vendors in the market in terms of the way they’re positioning what they offer? What is the kind of background to that kind of dislocation in the market?
Kevin: Yeah, if we’re focused on large corporations, in this case, they’re generally bad at moving quickly or adopting new things. Not because they’re not smart, but just there’s a lot of organizational obstacles to try new things. Particularly in a conservative industry. So, I think, in most cases, these companies are not digitally native. So, they have to learn a lot about AI. What is it looking to do? What are the different flavours of machine learning, supervised versus unsupervised? How does that matter for the problem I’m solving? And not all of them effectively are able to answer those questions early on, to point them in the right direction. So, I do think I think that there’s a lot of aspects of just education. Because if you go into any one of these organizations, you’ll find really cutting edge, really smart people who understand every nuance. There’s going to be people like that they are in these organizations. It is about them aligning these broader functions to execute together. That’s a really complicated problem in a big organization, because you have to get buy-in at the highest levels, all the way down throughout the organization down to the people who are doing the work, hands on, day to day. So, getting that kind of unity of vision, executing it together efficiently. It’s just hard. It’s hard, if anything, is particularly hard with a technology like AI that a lot of them might not be familiar with.
Ray: Okay. It fundamentally looks like it’s people, in its simplest format.
Kevin: Yeah, summing up it is people are — people are complicated.
Ray: So, just having some fun with it now. Imagine a world where on the data front, most industries have had their house in order, the people piece is aligned, data science capability is in place; what does utopia look like? What are some of the blue sky, really sexy opportunities out there on how machine learning, AI, computer vision, other form factors of AI can really transform the innovation process? What are some of the holy grails which you guys generally keep your eye on and really excite you?
Kevin: To me, exciting is, Can I make valuable products faster? And that sounds a little bit boring, but I think whatever industry you’re in, whether you’re product is a drug, or a coding, or a device, is Can I make a better version of this more valuable version to my customer? And can I do it fast? Because you think, at some point, there’s going to be a more level playing field, obviously, there’s going to be winners and losers and people who are better at it than others. But AI is going to be fairly democratized. I mean, anyone is going to be able to do it if they want. So, the ones who really continue successfully, translate those insights that come out of whatever data they put into their AI algorithm, to really just build interesting things that are more valuable. For me, as a consumer, for example, just having better things that have more utility to me, and have them come out more frequently or more often, or provide more value to me, I think that’s the thing we can hope for. I think it’s going to happen. It just depends on what sector, it depends on what industry. But if we if we truly envision a world where everyone can do this pretty well, we should get better stuff on the back end.
Ray: Do you see any macro tailwinds really fast-tracking how AI will impact that entire R&D process? So, for example, we’re seeing some really interesting developments on edge computing and, and also amazing businesses like Nvidia and the potential huge merger of ARM and Nvidia really creating that end -to-end stack when it comes to enabling AI at scale. That is just one example, but do you see other macro tailwinds which would really be a force multiplier to accelerate this vision of AI driven research and development?
Kevin: I think you pointed out an interesting one in edge computing. We’re not needing a ton of computational power and getting insights out of AI faster at the edge. I think that’s fascinating. In terms of macro tailwinds, I think that there’s generally an understanding that AI can be transformative, and that it’s coming. So, I do think you see governmental policies and regulations put into place or support for AI development. You certainly see that in China, for example. Some of these governments are starting to recognize the impact of the application of these technologies and will absolutely push this forward and help industries grow and, and will help motivate, I think the application of AI and R&D. So, I do think there’s not just market forces, there’s also regulatory forces that can also push these forward. But I think if you’re looking for a signal, you know, you go to LinkedIn, and just look up all the people with digital transformation in their title. These functions, these jobs, these things are popping up everywhere, and in all kinds of industries, not just for marketing, and not just in traditional tech. But companies that make pumps and companies that do a variety of different things, this understanding of how disruptive these technologies can be, are coming to just a variety of industries. So, I think you’re going to see it continue to proliferate.
Ray: Yeah, Kevin, I love your signal on doing that quick search on LinkedIn. We do that from time to time here at PatSnap. We did a search just literally on innovation, Kevin, and the numbers were stunning. If you were to benchmark them against other areas, like even areas like decision science or data science, it yielded some focused results. It got everyone just really excited about the impending wave. I think job creation is a great signal to potential software categories and technology categories. So, I like that one as an example. So, Kevin, let’s get into kind of that Disneyland, imaginative state. So, if we were sitting here in 2028, where do you think we would be on the scale on how AI has evolved the innovation process? Or where do you think we’ll be at in terms of impact?
Kevin: Yeah, I think if we go back to this, we think about the whole innovation process starting at that front end, where you’re ideating. I believe by 2020, you’ll see much more enterprise deployment of tools to actually do this robustly. If we’re in the early days now, like we talked about before, you should see maturity, you should see people learn from their past failures and we should see implementation of just better ways and more systematic ways of choosing what we work on next. I think I think it would be a disappointment if we didn’t see advances there. So, if you’re looking for tangible measures, you know, there’s measurements of the number of initiatives that turn into successful products, and it’s not good. If we look back, my anticipation and hope is that within the decade, you’ll start to see the hit rate or success rate of idea to value increase, however, that’s going to be measured, or how do we measure it today. We should see organizations start to see a true uptick in some kind of metric like that, because otherwise, then I’d say this experiment has been a failure.
Ray: That’s definitely a vision that we get really excited about as well. This whole piece around analytics driven innovation, where you’ve got a confluence of unstructured data all connected. Where do you think we are on that front, in terms of market understanding and acceptance on using a range of unstructured data, and linking that together to glean foresight. Where do you think we are in terms of development on that front?
Kevin: I’d say still in the early days of that, and I think the disconnect is, I suspect you and I could talk a long time about this Ray, is the belief that data itself will be the end solution. So, I think that the disconnect is really, you’re not really looking for data. That’s not the objective, the objective is the insight. You want something that can tell you what to do next. How do I take whatever I’m working on and make it more successful? So, where I see the disconnect is, you’ve got to come at this from two ends, you come at this from that data unification standpoint that you’re talking about and I think that’s rapidly improving a lot of cool stuff happening there. But I do think, coupling that with the human in the loop, the right person on the other end of that spectrum, to interpret the data, interpret those trends, to pull out that insight, and get you to whatever you’re supposed to do next. I think that’s the gap that needs to be closed, cutting edge data tools, cutting edge algorithms, trends, predictions, forecasts, coupled with people who can really make educated decisions based on those insights. I think that there’s room to join those two things more effectively. So, I think we’re making huge strides on all that, you know. We’ve got the experts in different places, the data is coming up, how do we join them and not make it necessarily a competition between them but make it more kind of a unified goal to make those things work better together.
Ray: So when you mentioned human in the loop deck, Kevin, are you touching upon where you might have market insights teams at certain companies or foresight teams being intimidated by potentially machine learning displacing some of their work? So, there’s that kind of cultural resistance? Or is that a challenge? Or is it just fundamentally understanding the technology? How do we bridge that holy grail gap of ML unstructured data and human in the loop working in synergy?
Kevin: I think it’s both of the things you mentioned. There’s certainly a threat, or threatening aspects, you only need to look up workforce automation, or AI and jobs of the future and see how much discussion there is about the fear of displacing jobs. Some jobs will be displaced, of course, but it is a natural reaction when you talk about automation, which is really a big push of what we’re talking about here: automating insights. Of course, that’s a threat to people whose job it is to generate and interpret insights, based on data. So, I think there’s definitely going to be that obstacle from just a threatening personal standpoint, you know, threatening the expertise of people. But where I truly believe in the human in the loop part of this, is that particularly if you work in an organization that is science-based, you will never trust an algorithm blindly. As a scientist myself, I would never, I don’t care how much it’s been explained to me, I don’t care how rigorously it’s been developed, a good scientist is always going to be skeptical about results presented to them. So, you’re always going to have to bridge that gap where hopefully you can superpower that innovator. You know, whether it’s a foresight person or a scientist, you know, my position I guess I would be on this, this set of technologies would be how do we use it to superpower the people, the smart people we already have, rather than displace them. I know that might be controversial, that might differ from other perspectives, but I believe that’s the vision that I would most embrace going forward.
Your recommended content
-
Innovations in the crypto space and the future of commercial Web3 adoption with Ken Chia
Friday, June 10, 2022
Ray is joined by Ken Chia, head of APAC for Abra, the world’s premier crypto wealth management platform. Ken made the leap from traditional finance into Web3 when he realized the exponential growth potential for this market. Follow along as Ray and Ken explore Abra, the stability regulations of the Web3 space, and possible market setbacks from inflation, the war in Ukraine, and the energy crisis.
-
Social Tokens and Creator Communities with Brian Mark, Director of Rally
Wednesday, May 18, 2022
Brian Mark, Director and Content Educator at Rally, chats with Ray about social tokens and how this form of co-created cryptocurrency creates a feeling of community on Web3.
-
Web3 Mobilizes Communities for Social Good, with Pat Kearney at Thirdweb
Tuesday, May 3, 2022
Ray is joined by Pat Kearney, Head of Growth at Thirdweb. Pat discusses the limitless potentialof Web3 to “mobilize and incentivize communities for social good and create positive impact.”Highlights